Main Points In Hindi (मुख्य बातें – हिंदी में)
-
AI का अंगीकरण: कृत्रिम बुद्धिमत्ता और मशीन लर्निंग कृषि और वानिकी जैसे क्षेत्रों में प्रौद्योगिकी को संचालन में दक्षता और सटीकता लाने के लिए लागू की जा रही है। स्वायत्त ट्रैक्टर और हारवेस्टर जैसे मशीनें मानव कार्य का बहुत अधिक हिस्सा स्वचालित कर रही हैं, जिससे उत्पादकता और संसाधनों का प्रबंधन बेहतर हो रहा है।
-
सामाजिक और पर्यावरणीय चिंताएँ: एआई-चालित मशीनरी से होने वाले लाभों के साथ-साथ यह भी चिंता है कि यह छोटे किसानों के लिए रोजगार अवसरों को नष्ट कर सकती है और उच्च लागत डिजिटल विभाजन को जन्म दे सकती है। इसके अलावा, अत्यधिक स्वचालन से वनों की कटाई में वृद्धि हो सकती है, जिससे पर्यावरण पर नकारात्मक प्रभाव पड़ सकता है।
-
सटीक खेती और संसाधन प्रबंधन: एआई-आधारित तकनीकें संसाधनों के अधिकतम उपयोग के लिए डेटा संग्रहित करती हैं, जिससे पानी, खाद और कीटनाशकों का प्रयोग कम होता है। इससे न केवल लागत में कमी आती है, बल्कि यह पर्यावरण संरक्षण में भी योगदान करती है।
-
पारंपरिक रखरखाव के लाभ: मशीन लर्निंग का उपयोग करके पूर्वानुमानित रखरखाव संभव हो गया है, जिससे मशीनरी के डाउनटाइम में कमी आती है और संचालन की निरंतरता बनाए रखी जाती है। उदाहरण स्वरूप, कैटरपिलर जैसे कंपनियाँ डेटा का विश्लेषण कर महत्वपूर्ण घटकों की विफलताओं की पूर्वावधि करती हैं।
- भविष्य की चुनौतियाँ: एआई-चालित तकनीकी प्रणालियों के आगे आने वाली चुनौतियाँ जैसे कि डेटा सुरक्षा, प्रशिक्षण की आवश्यकताएँ और विकासशील क्षेत्रों में अपनाने में बाधाएँ महत्वपूर्ण हैं। इन सबका समाधान ढूंढने के लिए प्रौद्योगिकी और नीति में सटीक दृष्टिकोण अपनाने की आवश्यकता है।
Main Points In English(मुख्य बातें – अंग्रेज़ी में)
Here are the main points derived from the provided text:
-
AI Revolution in Agriculture and Forestry: Artificial intelligence (AI) and machine learning are transforming agriculture and forestry by automating manual labor and improving efficiency, precision, and resource management. Technologies like autonomous tractors and AI-driven forestry machinery are leading these innovations, resulting in enhanced productivity and sustainability.
-
Benefits of AI Implementation: Key advantages of incorporating AI in heavy machinery include improved efficiency and higher crop yields, significant cost savings through optimized resource use, better safety by automating dangerous tasks, and enhanced decision-making through real-time data insights.
-
Challenges and Ethical Considerations: Despite the benefits, challenges such as high initial costs, the need for technical competencies, data security, and privacy concerns persist. Additionally, the potential social implications, including job displacement and access disparities for small farmers, raise ethical questions regarding AI adoption.
-
Predictive Maintenance and Resource Management: AI enables predictive maintenance of heavy machinery, reducing downtime and prolonging equipment life. Moreover, precision farming through AI allows for better resource management, minimizing waste and environmental impact while optimizing input applications like water and fertilizers.
- Future Trends: Future developments in AI for agriculture and forestry include the integration of drones and satellite imagery for monitoring crop health, the emergence of AI-powered robots for specialized tasks, and advancements in predictive analytics to improve operational planning and sustainability practices.
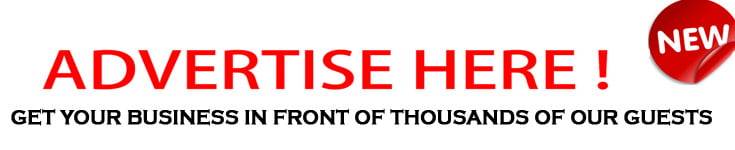
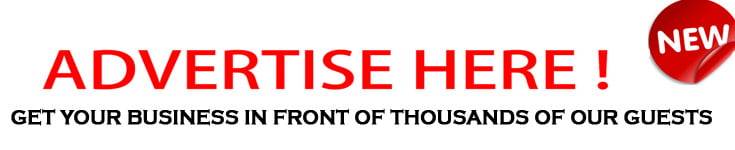
Complete News In Hindi(पूरी खबर – हिंदी में)
With growing demands on efficiency and precision, AI-driven heavy machinery or equipment is becoming a game-changer in enhancing productivity, sustainability, and resource management.
Revolutionizing Agriculture and Forestry with AI-Driven Heavy Equipment
Revolutionizing Agriculture and Forestry with AI-Driven Heavy Equipment
Shafqaat Ahmad, Data Scientist
Artificial intelligence and machine learning are becoming the face of sectors that needed much manual intervention and repetition earlier, such as agriculture and forestry. Nevertheless, with growing demands on efficiency and precision, AI-driven heavy machinery or equipment is becoming a game-changer in enhancing productivity, sustainability, and resource management. But despite technological promises, challenges remain for AI adoption in these sectors: social implications, regulatory issues, and accessibility constraints. The paper discusses applications related to AI in heavy machinery, advantages, challenges, and social and ethical considerations; it accounts for some case studies and future trends relevant to this sector.
AI Applications in Heavy Equipment
1. Autonomous Tractors and Harvesters: Driving Precision in Agriculture
Autonomous tractors and harvesters are reinventing agriculture by automating tasks that require immense human engagement. Such machines use sensors, cameras, GPS, and ML algorithms to navigate through fields, plant seeds, plow the soil, and harvest crops with unprecedented precision. At this point, an illustrative fact must be represented by the line of autonomous tractors developed by John Deere, utilizing deep-learning algorithms and computer vision to locate the precise position of crops regarding obstacles and optimize planting routes. The result would be less dissipation, more efficiency, and reduced labor costs.
Real-time data collection through sensors further enables the possibility of field conditions, including changes in topography and moisture levels. Autonomous combine harvesters can also be fitted with AI that assesses crop quality and moisture content to help further farmers make more informed decisions on when to harvest. This leads to better yields and lower costs due to lower fuel consumption and fewer human errors.
Social Implications and Ethical Concerns
While these developments promise efficiency, there are also apprehensions over the possible job displacement and accessibility issues for small farmers. Fully autonomous heavy equipment would drastically reduce the need for labor-intensive work. This might affect jobs in rural areas. The high cost may be another factor that creates a digital divide whereby only large-scale farms can access online farm management services.
2. AI-Driven Forestry Machinery: Enhancing Safety and Efficiency
It is a high-risk, heavy industry requiring toil in rugged terrain. The AI-led equipment, such as autonomous feller bunchers, forwarders, and harvesters, uses machinery vision, LiDAR sensors, and GPS to chart their independent way over the terrain, detect obstacles, and check tree conditions. The Ponsse Scorpion Harvester is one of the most imposing examples of using artificial intelligence in forest machines, which guarantees good logging and higher safety.
These machines can change their gestures and motions based on tree size, soil conditions, and terrain, all thanks to AI algorithms. Such machines would essentially automate high-risk jobs like filing and processing, reducing the chances of accidents and injuries. This further helps in the continuous monitoring of machinery health, thereby allowing predictive maintenance that improves operational safety and decreases costs about downtimes.
Ethical and Environmental Considerations
This would raise ethical questions regarding replacing human roles with high-risk tasks within regions where forestry provides critical employment. Further, while AI optimizes resource employment, the impact of wide-scale automation in such an environment cannot be overlooked. For example, if poorly managed, increased deforestation due to efficiency in the tree-cutting process might lead to more significant environmental degradation.
3. Precision Farming and Resource Management
Accordingly, large volumes of data from sensors, drones, and satellite imagery use AI to enhance precision farming practices greatly. The data optimizes resource application according to current crop and soil conditions about water, fertilizer, and pesticides. For example, an AI-powered irrigation system makes predictions for future water requirements using advanced machine learning models that consider forecasts related to weather conditions, current soil moisture levels in real-time, and stages of growth occurring within a crop.
With AI, heavy machinery can change operations dynamically, applying exact quantities of inputs to specific areas in the field. This precision is cost-effective and eco-friendly, minimizing the overuse of resources and reducing all kinds of pollution risks.
Regulatory and Policy Challenges
AI-based precision farming, therefore, raises regulatory issues on data privacy, standardization related to the equipment used in AI, and adherence to guidelines on the environment. An increased use of data raises questions about the ownership of the data and how the data is used. The policies must address such issues if the sector is to witness responsible and secure adoptions of AI.
4. Predictive Maintenance with Machine Learning
Heavy agricultural and forestry machinery often works in harsh conditions, which leads to wear and tear on the machine. AI has enabled predictive maintenance, hence reducing potential downtime and lowering costs. Algorithms of AI collect data from sensors mounted on machines to figure out patterns consistent with malfunctioning or deterioration of equipment.
For example, Caterpillar uses machine learning to analyze sensor data and predict critical component failures. This predictive maintenance prolongs the life of the machinery and prevents costly downtime. Predictive maintenance helps maintain productivity with minimum disruption in the forests, as an acre of lost trees causes substantial financial losses.
Benefits and Challenges of AI in Agriculture and Forestry
Benefits
- Improved Efficiency and Higher Yields: Automating labor-intensive tasks further increases the speed and precision of operations, improving yields and reducing waste. Besides, an autonomous piece of equipment also allows more area coverage in a much shorter time frame; various productivity rates increase manifold.
- Cost Savings: AI technology saves money by optimizing process operation, minimizing fuel usage, and reducing routine maintenance costs. Again, precision farming methods minimize the application costs of fertilizers and pesticides.
- Better Safety: Automation of potentially dangerous tasks, like in forestry, lessens the chances of accidents and injuries; hence, it makes workers safe and more efficient at work.
- Sustainability and Environmental Impact: These precision farming techniques optimize resource use, manage environmental pollution, and contribute towards sustainability. AI permits more efficient water, fertilizer, and pesticide management, conserving natural resources.
- Smarter Decision Making: AI avails real-time insights with actionable data to inform decision-making and even quicker reaction times to changing field conditions.
Challenges
- High Initial Costs: High costs involve the appropriate investments in technology, machinery, and workforce training to implement AI-driven heavy equipment. Smaller operations will not possibly be able to afford such high upfront fees.
- Techno-Competencies: AI integration will be successful if the professionals have the required data analysis, machine learning, and sensor technology competence. This gap needs enormous investment in education and training.
- Data Security and Privacy: Most AI-based systems require large datasets as inputs; thus, the data security and privacy question is pretty strong. Keeping sensitive data secure and using it ethically helps to build stakeholder trust.
- Barriers to Adoption in Developing Regions: The chances of adopting AI-driven heavy machinery in developing regions will remain limited due to a lack of awareness, resources, and basic infrastructure. Such barriers must be overcome through will and funding targeted by governments and organizations.
Case Studies of AI Adoption
John Deere’s Autonomous Equipment
John Deere manufactures most agricultural machinery by integrating AI into its autonomous tractors and combine harvesters. Advanced computer vision systems coupled with deep learning algorithms allow this equipment to identify crops, weeds, and obstacles with higher outcomes of improvement in planting routes for higher yields and optimal harvest times based on real-time data. It ensures higher crop yield with reduced labor costs and minimal environmental impact. However, small-scale farms also resist due to the high initial investment price for such advanced machinery.
ponsse’s Scorpion Harvester
Ponsse Scorpion Harvester is an excellent example of AI application in forestry. Because AI algorithms operate on real-time sensor data, this harvester can track challenging topography or soil and optimize the logging operation. This machine will vary the cutting and processing method according to tree size and ground conditions for greater efficiency with less environmental impact from forestry operations. On the other hand, if it is not regulated with proper policies, increased efficiency could lead to intensified deforestation.
Future Trends and Developments
1. Integration of Drones and Satellite Imagery
With high-resolution cameras and multispectral sensors, drones can capture images of crops and forests. Images captured can then be analyzed through artificial intelligence algorithms to determine the effects of diseases, deficiencies in nutrition, and pest infestation among crops or trees. Such integration provides real-time visibility into crop or forest health and density to manage the same proactively. For instance, AI-powered drones in agriculture assist farmers with crop health issues that need to be addressed promptly. In forestry, the health of trees, including forest density and fire hazards, can be monitored using drones.
2. AI-Powered Robots for Specialized Tasks
AI-powered robots will gradually emerge as solutions for specific tasks in agriculture and forestry. In agriculture, such robots can perform selected harvesting using ML algorithms that reduce plant damage. In the forestry sector, AI robots are being developed for tasks that include planting trees and selective logging. It is to be expected that such robots will negotiate rough terrain and work in hazardous conditions, thus enhancing levels of both safety and efficiency.
3. Advancements in AI-Driven Predictive Analytics
AI-driven predictive analytics models will improve decision-making related to agriculture and forestry. These models analyze historical weather data, forecasts, and market trends to indicate the probability of a condition occurring in the future, thus enabling operations planning. In turn, this allows AI to assist farmers with decisions on the best time to plant yeasts based on current weather and soil conditions. In the case of forestry, predictive models point to sustainable practices and help arrive at long-term climate change-related impacts on forest health.
Concrete Data and Statistics
Recent studies have shown that AI-driven precision farming increases crop yields by about 20-25%, reduces the costs of inputs such as water and fertilizers by 15-30%, and improves planting. Similarly, In forestry, accident rates could be reduced by up to 40% just by AI equipment. Regarding this, the technology ousts safety concerns over accidents and provides enormous operational efficiency. Predictive maintenance strategies could decrease downtime by 25% with significant cost savings in both industries.
Conclusion
AI-operated machinery will continue transforming agriculture and forestry into increasingly efficient, sustainable, and profitable industries. In other words, practical examples include autonomous tractors, AI-operated forestry machinery, and also precision farming techniques. Conversely, high costs, technical expertise, and data privacy make this challenging. Further, automation, which reduces human labor through machines, carries social implications and regulatory frameworks for responsible AI adoption.
More scope for innovation in agriculture and forestry will be accorded with each passing day by the ongoing AI revolution: drones, robots, and predictive analytics-installing a new vista in optimizing operations and managing resources optimally can be guaranteed. Yet, the ethical, environmental, and social dimensions of such AI adoption need to be weighed by stakeholders with due diligence. It is here that substantial investment in AI technologies, yet using them responsibly, will open ways for leading these industries to an intelligent and efficient future.
The content & opinions in this article are the author’s and do not necessarily represent the views of AgriTechTomorrow
Comments (0)
This post does not have any comments. Be the first to leave a comment below.
Featured Product
Elmo Motion Control – The Platinum Line, a new era in servo control
Significantly enhanced servo performance, higher EtherCAT networking precision, richer servo operation capabilities, more feedback options, and certified smart Functional Safety. Elmo’s industry-leading Platinum line of servo drives provides faster and more enhanced servo performance with wider bandwidth, higher resolutions, and advanced control for better results. Platinum drives offer precise EtherCAT networking, faster cycling, high synchronization, negligible jitters, and near-zero latency. They are fully synchronized to the servo loops and feature-rich feedback support, up to three feedbacks simultaneously (with two absolute encoders working simultaneously). The Platinum Line includes one of the world’s smallest Functional Safety, and FSoE-certified servo drives with unique SIL capabilities.
Complete News In English(पूरी खबर – अंग्रेज़ी में)
As industries seek greater efficiency and precision, AI-powered heavy machinery is changing the game by boosting productivity, promoting sustainability, and improving resource management.
Transforming Agriculture and Forestry with AI-Enabled Heavy Equipment
Transforming Agriculture and Forestry with AI-Enabled Heavy Equipment
Shafqaat Ahmad, Data Scientist
Artificial intelligence (AI) and machine learning are taking the lead in sectors like agriculture and forestry that traditionally relied heavily on manual labor. AI-driven machines are proving to be essential in improving efficiency, sustainability, and the management of resources. However, despite the technological advancements, challenges such as social implications, regulations, and access to these technologies still exist. This paper explores AI applications in heavy machinery, along with their benefits, challenges, and ethical considerations, supported by case studies and future trends in these sectors.
AI Applications in Heavy Equipment
1. Self-Driving Tractors and Harvesters: Precision in Agriculture
Self-driving tractors and harvesters are transforming agriculture by automating tasks that usually require extensive human effort. These machines are equipped with sensors, cameras, GPS, and machine learning algorithms to navigate fields, plant seeds, till the soil, and harvest crops with exceptional accuracy. For example, John Deere’s line of self-driving tractors employs deep learning algorithms and computer vision to pinpoint crop locations relative to obstacles, optimizing planting paths and leading to reduced waste, increased efficiency, and lower labor costs.
Through real-time data collection, these machines assess field conditions, including changes in terrain and moisture levels. Self-driving harvesters can analyze crop quality and moisture content, helping farmers decide the best time to harvest. This results in better yields and lower operational costs due to reduced fuel consumption and fewer human errors.
Social Implications and Ethical Concerns
While AI promises improved efficiency, there are concerns about job displacement and accessibility for small farmers. Fully automated machines could significantly decrease the need for manual labor, impacting employment in rural areas. Additionally, the high cost of technology may create a digital divide, restricting access to large-scale farms that can afford such advanced equipment.
2. AI-Driven Forestry Machinery: Improving Safety and Efficiency
Forestry is a challenging industry, often involving risky work in difficult terrains. AI-powered machines, such as autonomous feller bunchers, forwarders, and harvesters, use machine vision, LiDAR sensors, and GPS to navigate their surroundings, identify obstacles, and assess tree conditions. One notable example is the Ponsse Scorpion Harvester, which leverages AI for efficient logging while enhancing safety.
These machines can adjust their movements based on tree size, soil type, and terrain conditions, all thanks to AI. This automation reduces the risks associated with dangerous tasks, lowers the likelihood of injuries, and allows for continuous monitoring of the machinery’s health, leading to more effective maintenance and reduced downtime costs.
Ethical and Environmental Considerations
However, there’s an ethical dilemma regarding the replacement of human jobs in forestry, which provides vital employment in many regions. While AI can enhance resource management, the risk of increased deforestation due to the efficiency of tree-cutting processes could lead to significant environmental harm if not carefully managed.
3. Precision Farming and Resource Management
AI greatly enhances precision farming by utilizing data from sensors, drones, and satellite images. This data helps in applying resources like water, fertilizers, and pesticides more accurately according to the specific needs of the crops and soil. For instance, an AI-powered irrigation system estimates future water needs by considering weather forecasts, current soil moisture levels, and crop growth stages.
With AI, heavy machinery can adapt its operations to apply exact amounts of resources to specific areas in fields, which is both cost-effective and environmentally friendly, reducing resource waste and pollution risks.
Regulatory and Policy Challenges
The rise of AI in precision farming also brings regulatory challenges concerning data privacy, equipment standards, and environmental compliance. As data usage grows, questions arise regarding data ownership and management. Addressing these issues through effective policies is crucial for responsible and secure AI adoption in the sector.
4. Predictive Maintenance Using Machine Learning
Heavy machinery in agriculture and forestry often operates in tough conditions, leading to wear and tear. AI enables predictive maintenance, reducing downtime and costs. By analyzing data from sensors, AI can identify patterns indicating potential failures or deterioration of equipment.
For example, Caterpillar employs machine learning to interpret sensor data and forecast critical component failures, extending machinery life and preventing costly breakdowns. Predictive maintenance is vital for maintaining productivity, as lost trees from downtime can lead to significant financial losses.
Benefits and Challenges of AI in Agriculture and Forestry
Benefits
- Improved Efficiency and Higher Yields: Automating labor-heavy tasks increases the speed and accuracy of operations, resulting in better yields and less waste. Autonomous equipment also covers more ground in less time.
- Cost Savings: AI optimizes operations, cuts fuel usage, and reduces maintenance costs, while precision farming methods lower application costs for fertilizers and pesticides.
- Enhanced Safety: Automating hazardous tasks in forestry reduces the risk of accidents, improving worker safety and efficiency.
- Sustainability and Positive Environmental Impact: Precision farming techniques optimize resource use, reducing environmental pollution and promoting sustainability.
- Better Decision-Making: AI provides real-time insights and actionable data, enabling quicker responses to changing field conditions.
Challenges
- High Initial Costs: Implementing AI-driven machinery involves substantial investments in technology and training, which may be unaffordable for smaller operations.
- Technology Competence: Successful AI integration requires skilled professionals with knowledge in data analysis and machine learning, necessitating significant investment in education and training.
- Data Security and Privacy: AI systems typically rely on large datasets, highlighting the need for robust data security measures to maintain trust among stakeholders.
- Barriers in Developing Regions: Limited awareness, resources, and infrastructure hinder the adoption of AI-driven machinery in developing areas, necessitating support from governments and organizations.
Case Studies of AI Adoption
John Deere’s Autonomous Equipment
John Deere incorporates AI in its autonomous tractors and combine harvesters, where advanced computer vision and deep learning algorithms improve crop and weed identification. This technology enhances planting efficiency and harvest timing, boosting yields while cutting labor costs and minimizing environmental harm. However, small-scale farms often resist due to the high initial investment required for such machines.
Ponsse’s Scorpion Harvester
The Ponsse Scorpion Harvester exemplifies AI use in forestry by leveraging real-time sensor data to navigate challenging terrains and optimize logging operations. The machine adapts its cutting methods to tree size and ground conditions, promoting efficiency while minimizing environmental impact. However, without proper regulations, increased efficiency could lead to greater deforestation.
Future Trends and Developments
1. Using Drones and Satellite Imagery
Drones equipped with high-resolution cameras and sensors can analyze crop and forest health in real-time. This integration helps identify issues like diseases, nutrient deficiencies, and pest infestations, allowing farmers and foresters to manage their resources proactively.
2. AI-Powered Robots for Specialized Tasks
AI-driven robots are expected to take on specific tasks in agriculture and forestry. In farming, these robots can selectively harvest crops with minimal damage. In forestry, AI robots may perform tasks like tree planting and selective logging, improving safety and efficiency.
3. Advances in AI-Powered Predictive Analytics
Predictive analytics models driven by AI will enhance decision-making in agriculture and forestry, enabling better planning based on historical weather data and market trends. These models can help farmers determine optimal planting times while guiding foresters to adopt sustainable practices.
Data and Statistics
Recent research shows that AI-driven precision farming can increase crop yields by 20-25%, reduce input costs for water and fertilizers by 15-30%, and improve planting efficiency. In forestry, accident rates may decrease by up to 40% with AI equipment, addressing safety concerns while enhancing operational efficiency. Predictive maintenance can also lead to a 25% reduction in downtime, yielding significant cost savings across both industries.
Conclusion
AI-powered machinery is reshaping agriculture and forestry, making them more efficient, sustainable, and profitable. Examples include autonomous tractors, AI-driven forestry machinery, and precision farming techniques. However, challenges such as high costs, the need for technical expertise, and data privacy concerns persist. Social implications, including job displacement and the need for regulatory frameworks, must also be addressed. The ongoing AI revolution offers opportunities for innovation through drones, robots, and predictive analytics, but stakeholders must carefully consider ethical, environmental, and social issues as they adopt these technologies responsibly.
The views expressed in this article are those of the author and do not necessarily reflect the opinions of AgriTechTomorrow.
Comments (0)
This post does not have any comments. Be the first to leave a comment below.
Featured Product
Elmo Motion Control – The Platinum Line, a New Era in Servo Control
Elmo’s Platinum Line of servo drives offers significant enhancements in performance, precision networking, and operational capabilities. With faster response times and multiple feedback options, these drives ensure optimal performance while being compact and featuring advanced safety certifications.
This rewritten content simplifies the original text into easy-to-understand English while maintaining the fundamental ideas and details.
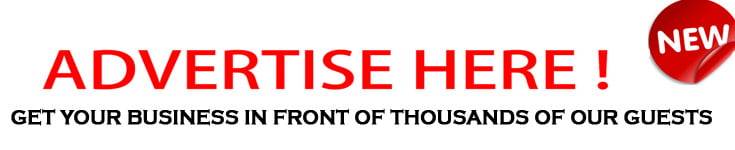
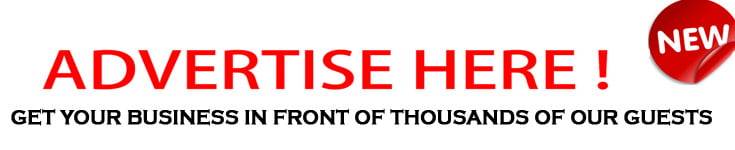